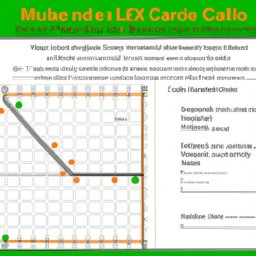
Monte Carlo Simulation
Page 34 | Listen in audio
Monte Carlo Simulation is a technique used in several areas, such as finance, engineering, physics, among others, to model complex systems and make predictions based on probabilities. This technique consists of generating a large number of random simulations of a model, based on probability distributions, and analyzing the results obtained.
In Excel, it is possible to use the Data Analysis tool to perform the Monte Carlo Simulation. For this, it is necessary to have a model that can be parameterized and that has one or more random variables. These variables can be defined using probability distribution functions, such as Normal, Uniform, Exponential, among others.
To perform the Monte Carlo Simulation in Excel, it is necessary to follow some steps. First, it is necessary to define the random variables and their respective probability distributions. Next, it is necessary to create a table with the expected results for each combination of random variable values. This table is known as the results table.
With the result table created, it is possible to use the Data Analysis tool to generate random simulations. To do so, simply select the "Monte Carlo Simulation" option and inform the number of simulations to be carried out. Excel will generate a set of random values for each defined random variable and, based on these values, will calculate the expected results for each simulation.
After carrying out the simulations, it is possible to analyze the results obtained and extract important information about the model. For example, it is possible to calculate the mean, standard deviation and other statistical indicators for the results obtained in all simulations. This information can be used to make more informed decisions and to assess the risks associated with the model.
In short, Monte Carlo Simulation is a powerful and versatile technique that can be used in many areas to model complex systems and make predictions based on probabilities. In Excel, it is possible to use the Data Analysis tool to perform the Monte Carlo Simulation in a simple and efficient way, as long as the random variables and their probability distributions are defined correctly.
Now answer the exercise about the content:
_What is the first step to carry out the Monte Carlo Simulation in Excel?
You are right! Congratulations, now go to the next page
You missed! Try again.
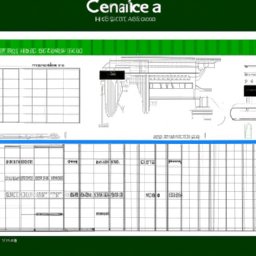
Next page of the Free Ebook: